The RTX 6000 ADA is a wildcard choice for any AI training and inference project. While it lacks multi-GPU connectivity of datacenter-focused GPUs, the ADA has a number of features that make it a solid choice for many AI initiatives.
Let’s review the RTX 6000 ADA’s key capabilities and compare its use cases against other high-performance GPUs available on the market such as the A6000, A100, and H100. This comparison helps you understand where the RTX 6000 ADA stands in NVIDIA's current GPU lineup and how it could benefit your next AI projects.
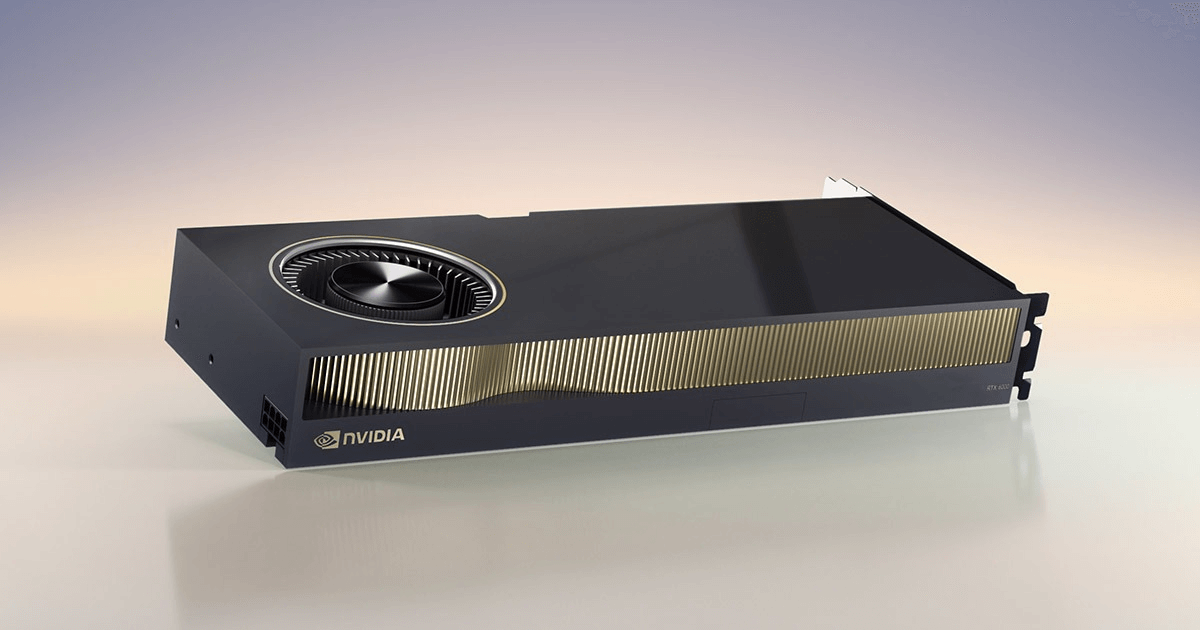
Overview of the NVIDIA RTX 6000 ADA GPU
The RTX 6000 ADA is a powerful workstation graphics card released by NVIDIA in 2022. While it shares some design components with NVIDIA gaming graphics cards such as the NVIDIA RTX 4090, it is purposely designed for high-performance workstation computing in a professional environment.
The NVIDIA RTX 6000 ADA is built on the Ada Lovelace architecture. It includes 18,176 CUDA Cores and 568 fourth-generation Tensor Cores, providing strong performance for AI tasks. Additionally, it includes 142 third-generation RT Cores, which is especially useful for workloads such as real-time rendering and complex simulations.
With a single-precision performance of 91.1 TFLOPS, the RTX 6000 ADA is among the most powerful workstation GPUs in AI performance. It comes with 48 GB of GDDR6 ECC memory and offers a decent memory bandwidth of 960 GB/s. The GPU has a relatively low power consumption of 300W and uses PCIe Gen 4.
This GPU is designed primarily for AI researchers, data scientists, and professionals who need powerful workstation capabilities. In this role, it is most often compared to the A6000. It is useful to also benchmark against key A100 specs and H100 specs.
Comparison Table of Key Specifications: A6000, RTX 6000 ADA, A100 & H100
Specification | NVIDIA A6000 | NVIDIA A100 | NVIDIA RTX 6000 ADA | NVIDIA H100 |
---|---|---|---|---|
Architecture | Ampere | Ampere | Ada Lovelace | Hopper |
CUDA Cores | 10,752 | 6,912 | 18,176 | 14,592 |
Tensor Cores | 336 (3rd Gen) | 432 (3rd Gen) | 568 (4th Gen) | 456 (4th Gen) |
Single-Precision Performance | 38.7 TFLOPS | 19.5 TFLOPS | 91.1 TFLOPS | 67 TFLOPS |
Tensor (FP16) Performance | 309 TFLOPS | Up to 624 TFLOPS | Up to 1457 FLOPS | Up to 1978 FLOPS |
Memory | 48 GB GDDR6 ECC | 40 GB or 80 GB HBM2e | 48 GB GDDR6 ECC | 80 GB HBM3 |
Memory Bandwidth | 768 GB/s | 1,555 GB/s | 960 GB/s | 3,000 GB/s |
Power Consumption | 300W | Up to 400W | 300W | Up to 700W |
Interconnect | PCIe Gen 4 | PCIe Gen 4 (PCIe variant), NVLink | PCIe Gen 4 | PCIe Gen 5 (PCIe variant), NVLink |
NVLink Support | For 2x A6000s | Yes | No | Yes |
Target Environment | Workstation | Data Center | Workstation | Data Center |
Multi-GPU Scalability | Moderate | High | Limited | High |
Release Year | 2020 | 2020 | 2022 | 2022 |
For additional GPU comparisons, see A100 vs H100 and L40S vs A100 and H100.
Comparing RTX 6000 ADA with NVIDIA A6000
The RTX 6000 ADA is based on the Ada Lovelace architecture, while the A6000 uses the Ampere architecture. This upgrade in architecture gives it several advantages in performance and efficiency.
Performance Metrics
The RTX 6000 ADA has 18,176 CUDA Cores compared to the A6000's 10,752 CUDA Cores. This difference translates to a significant increase in computational power. The RTX 6000 ADA delivers 91.1 TFLOPS of single-precision performance, whereas the A6000 provides 38.7 TFLOPS.
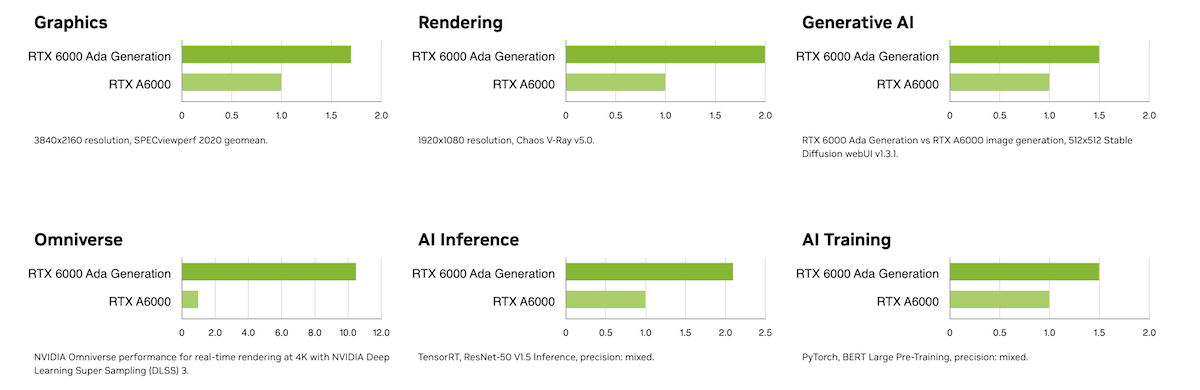
In terms of Tensor Performance, the RTX 6000 ADA offers up to 1.45 PFLOPS, while the A6000 reaches up to 1.25 PFLOPS. This makes the RTX 6000 ADA more suitable for AI training and inference tasks that require extensive tensor computations.
Memory and Bandwidth
Both GPUs feature 48 GB of GDDR6 ECC memory. However, the RTX 6000 ADA has a higher memory bandwidth of 960 GB/s compared to the A6000's 768 GB/s. This increased bandwidth allows for faster data transfer and improved performance in memory-intensive applications.
Power Efficiency
Both GPUs consume 300W of power. However, the RTX 6000 ADA’s architecture allows for a far better performance per watt, making it more efficient in terms of computational power relative to energy consumption.
RTX 6000 ADA vs A6000 Summary
The RTX 6000 ADA is ideal for high-end AI training, complex simulations, and real-time rendering. Its advanced features make it suitable for professionals who need top-tier performance in a workstation environment. On the other hand, the A6000 is adequate for tasks such as 3D rendering, video editing, and mid-level AI training.
Comparing RTX 6000 ADA with NVIDIA A100
Another common point of comparison is between the RTX 6000 ADA and the NVIDIA A100. The RTX 6000 ADA is a workstation GPU, while the A100 is designed primarily for datacenters. This fundamental difference influences their architecture and capabilities.
Performance Metrics
The RTX 6000 ADA has 18,176 CUDA Cores, significantly more than the A100's 6,912 CUDA Cores. This gives the RTX 6000 ADA an edge in raw computational power. Its single-precision performance is 91.1 TFLOPS, compared to the A100’s 19.5 TFLOPS. However, the A100 is optimized for mixed-precision and tensor operations, providing up to 624 TFLOPS of tensor performance.
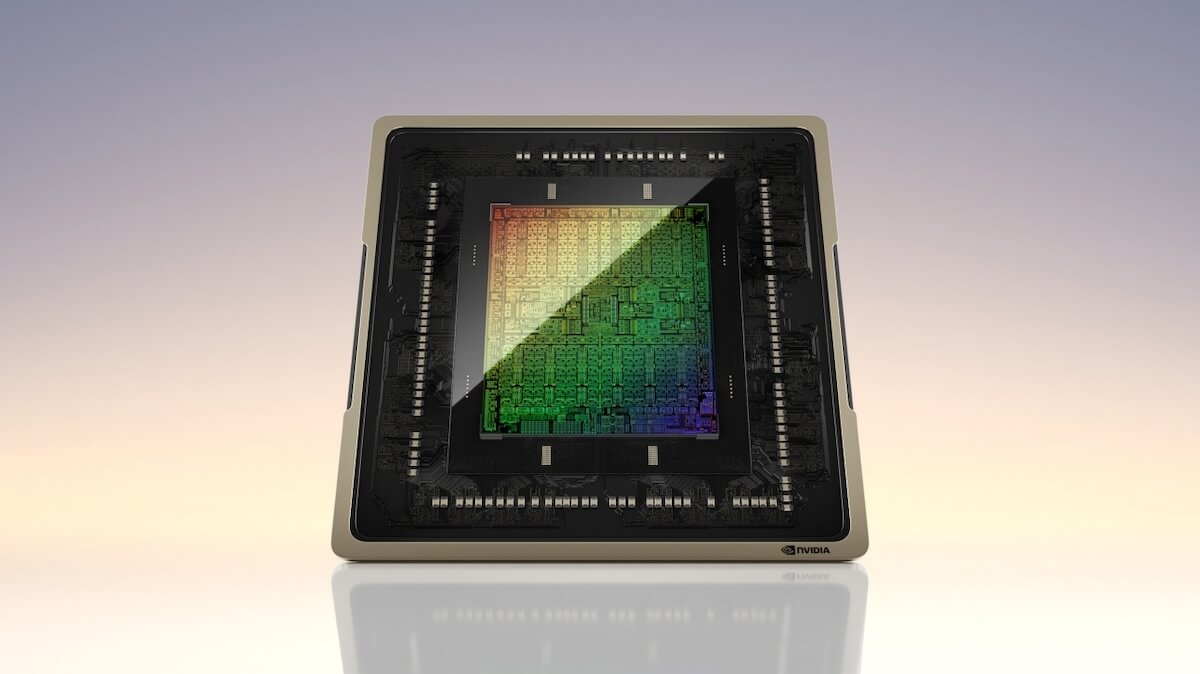
The RTX 6000 performance advantage comes from the powerful Ada Lovelace GPU architecture.
Memory and Bandwidth
The RTX 6000 ADA features 48 GB of GDDR6 ECC memory with a bandwidth of 960 GB/s. The A100 offers two variants: 40 GB or 80 GB of HBM2e memory, with a significantly higher bandwidth of 1,555 GB/s. This higher memory bandwidth supports larger models and datasets, crucial for advanced AI and ML tasks.
NVLink and Scalability
One of the major differences is the lack of NVLink support in the RTX 6000 ADA. The A100 supports NVLink, offering up to 600 GB/s GPU-to-GPU interconnect bandwidth. This makes the A100 highly scalable and suitable for large-scale AI training setups requiring multiple GPUs.
RTX 6000 ADA vs A100 Summary
The RTX 6000 ADA is best for high-performance computing tasks in workstations, including AI training and inference, real-time rendering, and complex simulations. The A100 is optimal for large-scale AI training and inference in data centers. Its scalability and memory capabilities make it ideal for enterprises requiring extensive computational resources.
Comparing RTX 6000 ADA with NVIDIA H100
The NVIDIA H100, built on the Hopper architecture, is the current top choice for large-scale AI deployments. It offers unprecedented performance with up to 1978 TFLOPS tensor processing, 80 GB of HBM3 memory, and 3,000 GB/s bandwidth. Most importantly, it has massive multi-GPU scalability in a datacenter setting thanks to NVLink technology.
While the RTX 6000 ADA, based on Ada Lovelace architecture, provides robust performance for single workstation tasks, but it lacks the scalability and extreme memory bandwidth of the H100, making the H100 superior for the most demanding AI applications in data centers.
Bottom line on the RTX 6000 ADA
The NVIDIA RTX 6000 ADA GPU gives substantial advantages over the A6000, with more CUDA Cores, higher TFLOPS, and better tensor performance. Compared to the A100 and H100, it excels in workstation environments but lacks the scalability and memory capabilities required for large-scale datacenter deployments.
You can choose between fixed- and dynamic pricing when deploying RTX 6000 ADA instances on the DataCrunch Cloud Platform.
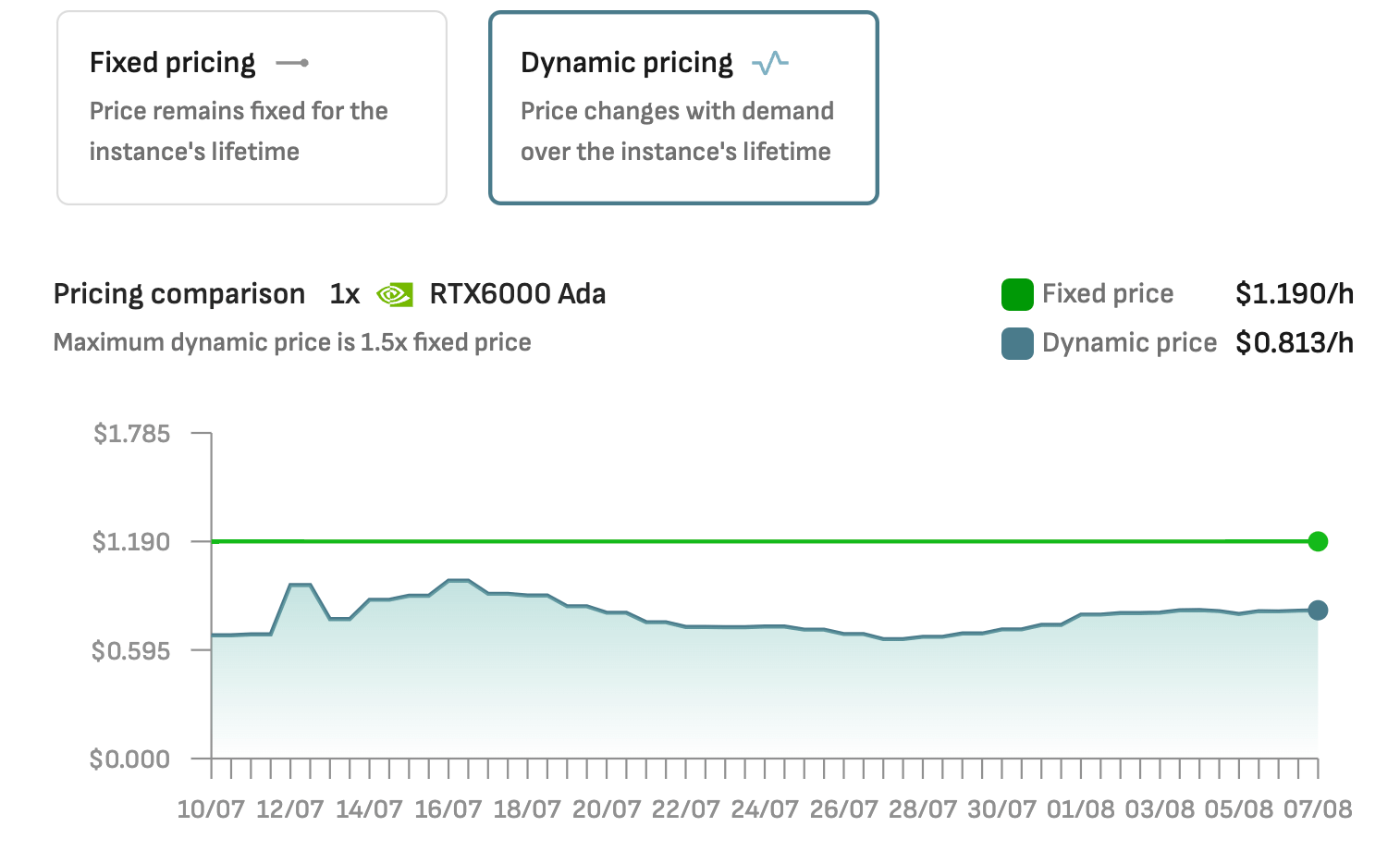
For AI researchers and professionals needing high-performance computing in workstations, the RTX 6000 ADA is still an excellent choice today. It can be capable of efficient AI training, inference, and complex simulations.
To see what you can do with the RTX 6000 ADA spin up an instance on the DataCrunch Cloud Platform today.