Choosing the right GPU is one of the most important decisions you can make when you’re starting a new deep learning project.
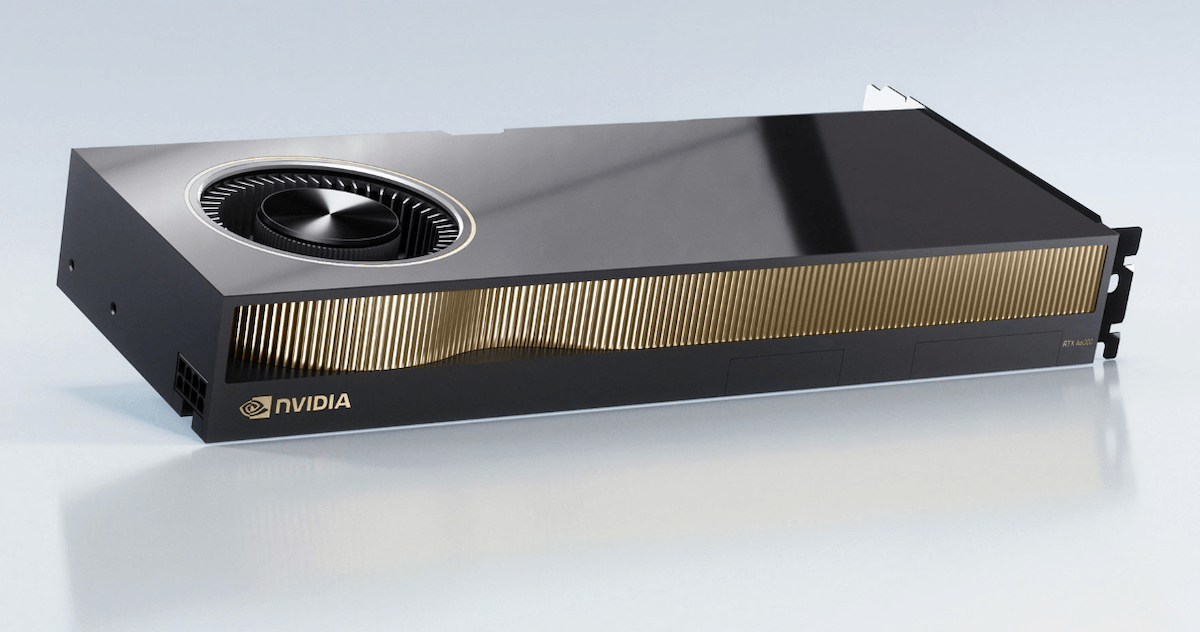
Only a couple of years ago, the RTX A6000 would have been a safe bet. Today this GPU still has uses, but you need to be aware of newer alternatives like the RTX 6000 Ada or the H100.
Why would you consider A6000 for deep learning projects?
In 2020 the NVIDIA RTX A6000 was a top pick for deep learning projects. With 48GB of VRAM it offered plenty of power to tackle demanding ML tasks – and it gave other workstation GPUs like the RTX 3090 a solid option for high performance computing.
The NVIDIA RTX A6000 was launched in October, 2020 as part of NVIDIA’s professional workstation lineup. Based on Ampere GPU architecture, it brought significant improvements over its predecessor, the RTX 6000, such as increased CUDA Cores and the introduction of the third-generation Tensor Cores. The outcome was the promise of up to 3.2x better TF32 AI training than the RTX 6000.
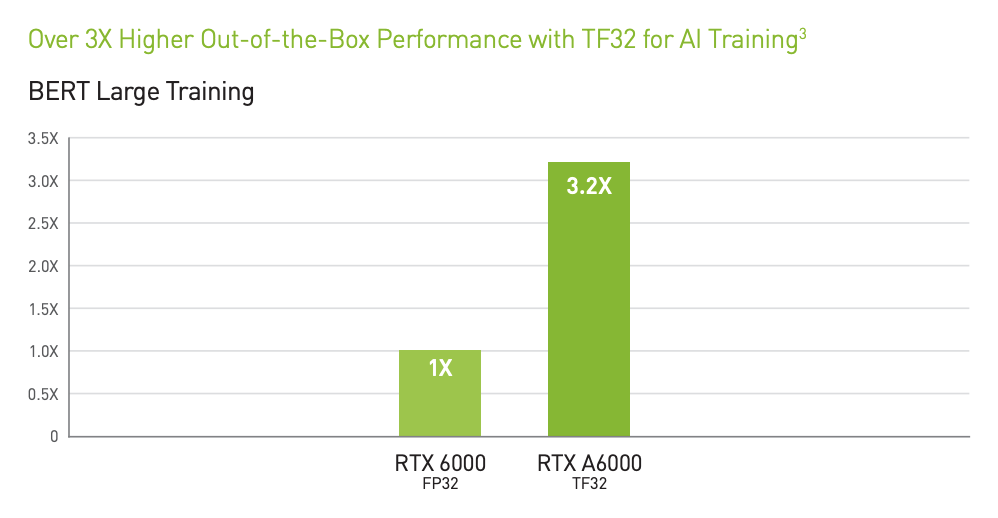
At the time of its release, NVIDIA positioned the RTX A6000 as the go-to workstation GPU for professionals working in AI, data science, and high-performance computing. It was promoted as ideal for demanding workloads such as training large neural networks, conducting complex simulations, and managing vast datasets. The combination of 48 GB of GDDR6 memory and 768 GB/s memory bandwidth made it particularly suitable for tasks requiring both raw performance and high memory capacity.
Shift to Datacenter GPUs
Today the game has changed in AI training and inference. The raw computing power needed for training large AI models has increased considerably. As a result, NVIDIA has released newer, more powerful GPUs like the RTX 6000 Ada and the H100. These new models give better price-per-watt performance, faster processing, and more advanced networking features tailored for AI.
This raises the question: Is the RTX A6000 still a good choice, or is it time to move on to something newer? Let’s answer this question by first looking at the specs.
Comparing the Specs: RTX A6000 vs. RTX 6000 Ada vs. H100
Below, we’ll compare the RTX A6000, RTX 6000 Ada, and H100 in terms of key specifications: CUDA Cores, Tensor Cores, memory performance, and price.
GPU Specifications Overview
Specification | RTX A6000 | RTX 6000 Ada | H100 |
---|---|---|---|
Architecture | Ampere | Ada Lovelace | Hopper |
CUDA Cores | 10,752 | 18,176 | 16,896 |
Tensor Cores | 336 | 568 | 528 |
RT Cores | 84 | 142 | N/A (Specialized cores) |
Memory | 48 GB GDDR6 | 48 GB GDDR6X | 80 GB HBM3 |
Memory Bandwidth | 768 GB/s | 960 GB/s | 2,048 GB/s |
Power Consumption | 300W | 350W | 700W |
Price-per-hour (Approx.) | $1.01/h | $1.19/h | $3.17/h |
*See more detailed specs for RTX 6000 Ada and H100.
CUDA Cores and Tensor Cores
The RTX 6000 Ada nearly doubles the CUDA cores compared to the RTX A6000, offering 18,176 cores. This increase translates to faster computations and better performance in tasks like deep learning and data analysis.
Tensor Cores are also key for AI-specific operations, such as matrix multiplications. The RTX 6000 Ada again outshines the RTX A6000 with 568 Tensor Cores compared to the A6000’s 336. If you’re working with large AI models or need to accelerate training, this is a significant upgrade.
The H100, with its 528 Tensor Cores, sits between the two in this respect but offers specialized cores designed specifically for AI at scale. The major difference is that the H100 has far better network capabilities, meaning that multi-GPU instances and clusters of H100s offer far superior performance to both the RTX A6000 and the RTX 6000 ADA.
Memory and Bandwidth
Memory performance is another critical factor, especially when working with large datasets or complex models. Both the RTX A6000 and RTX 6000 Ada come with 48 GB of memory, but the Ada model uses GDDR6X, which is faster and more efficient than the GDDR6 in the A6000. The memory bandwidth on the RTX 6000 Ada is also higher, at 960 GB/s compared to the A6000’s 768 GB/s, meaning faster data transfer and processing.
The H100 takes things to another level with 80 GB of HBM3 memory with a massive 2,048 GB/s bandwidth. This makes the H100 ideal for extremely data-intensive tasks, like training massive models or running simulations that require a lot of memory and fast access to it.
Power Consumption
The RTX A6000 is relatively power-efficient, drawing 300W, making it a more suitable option if energy consumption is a concern. The RTX 6000 Ada requires slightly more power at 350W, but it also delivers significantly better performance-per-Watt.
The H100, on the other hand, is a power-hungry beast, consuming 700W. This is a GPU designed for datacenters and large-scale AI operations where power consumption is less of a concern compared to raw computational power. Still, on an absolute basis, the H100 offers good performance-per-Watt efficiency.
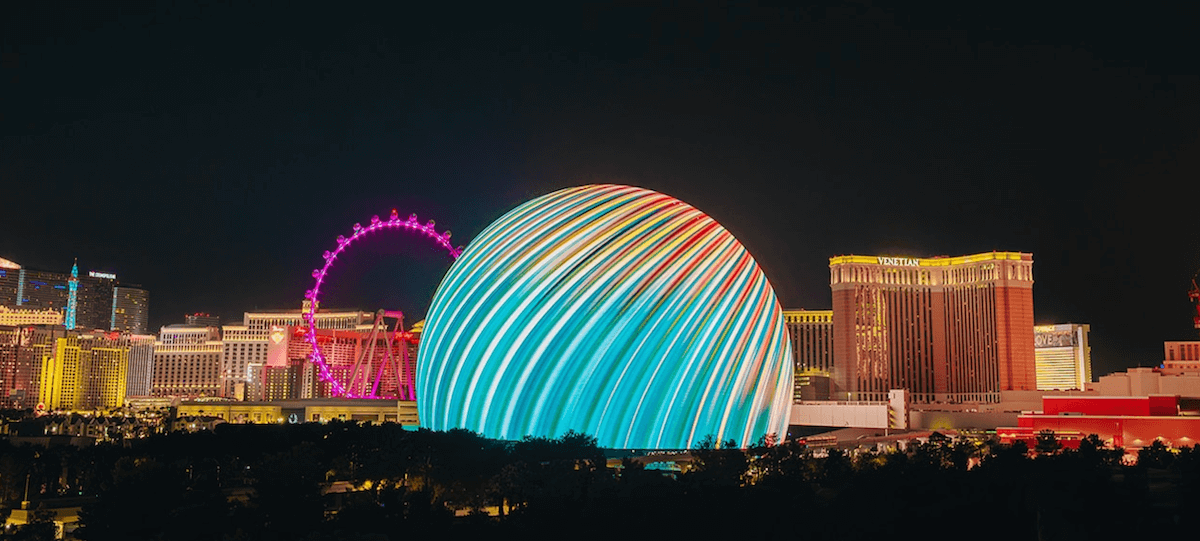
One notable use of the RTX A6000 is the Las Vegas Sphere. According to NVIDIA about 150 A6000’s are used to power up the visuals on the Sphere’s 16x16K displays and 1.2 million LED pucks at a 16K resolution at 60 frames per second.
TFLOPS Comparison
Ultimately, the capabilities of the A6000 are better compared in floating-point operations per second (FLOPS). Here is how it stands compared to the Ada and the SXM version of the H100.
Specification | RTX A6000 | RTX 6000 Ada | H100 (SXM) |
---|---|---|---|
Single-Precision (FP32) | 38.7 TFLOPS | 91.1 TFLOPS | 67 TFLOPS |
Half-Precision (FP16) | 309.7 TFLOPS | 1457 TFLOPS | 1979 TFLOPS |
RTX A6000: The RTX A6000 delivers 38.7 TFLOPS for single-precision floating-point operations (FP32). With its Tensor Cores, optimized for AI workloads, the A6000 can achieve up to 309.7 TFLOPS using mixed-precision (FP16) operations. Source: nvidia.com.
RTX 6000 Ada: The RTX 6000 Ada achieves up to 91.1 TFLOPS in FP32 calculations. With Tensor Cores the RTX 6000 Ada can reach 1,456 TFLOPS with FP16 operations. Source: nvidia.com.
H100 SXM: The H100 delivers 67 TFLOPS in Single-Precision FP32. In FP16 the H100 achieves 1979 with Tensor Cores and sparcity. Source: nvidia.com.
When to choose the RTX A6000
The RTX A6000 is okay for handling deep learning workloads. It doesn’t have the speed and power of the RTX 6000 Ada or H100, but it can handle many smaller AI model training and inference tasks efficiently.
Here are some scenarios where the RTX A6000 might be your best bet:
Medium-Scale Model Training:
If you’re working on training models that are large but not massive, the RTX A6000’s 10,752 CUDA cores and 336 Tensor Cores can be enough for your needs. As an NVIDIA GPU the A6000 can handle most deep learning frameworks efficiently, making it suitable for tasks like image recognition, natural language processing, and more.
AI Inference and Deployment:
When it comes to deploying trained models for inference—especially in environments where power consumption and cost are concerns—the RTX A6000 is an excellent option. It offers good performance while being more power-efficient and cost-effective compared to newer models.
General-Purpose Computing:
Beyond AI, the RTX A6000 is also versatile enough for other GPU-intensive tasks like video rendering, 3D modeling, and simulations. This makes it a good choice as a workstation GPU if your work involves a mix of AI and other compute-heavy applications.
Good availability and low-cost as Cloud GPU
While the price of the RTX A6000 has decreased considerably since 2020 it probably isn’t a smart idea to buy this GPU today for your workstation. There are more recent GPUs that can offer a better total-cost-of-ownership (TCO) over the lifetime of the device.
The good news is that the A6000 is relatively well available on Cloud GPU platforms such as DataCrunch, so renting the GPU is easy. The cost-per-hour you’ll pay for accessing this GPU is likely to be lower than other similar alternatives.
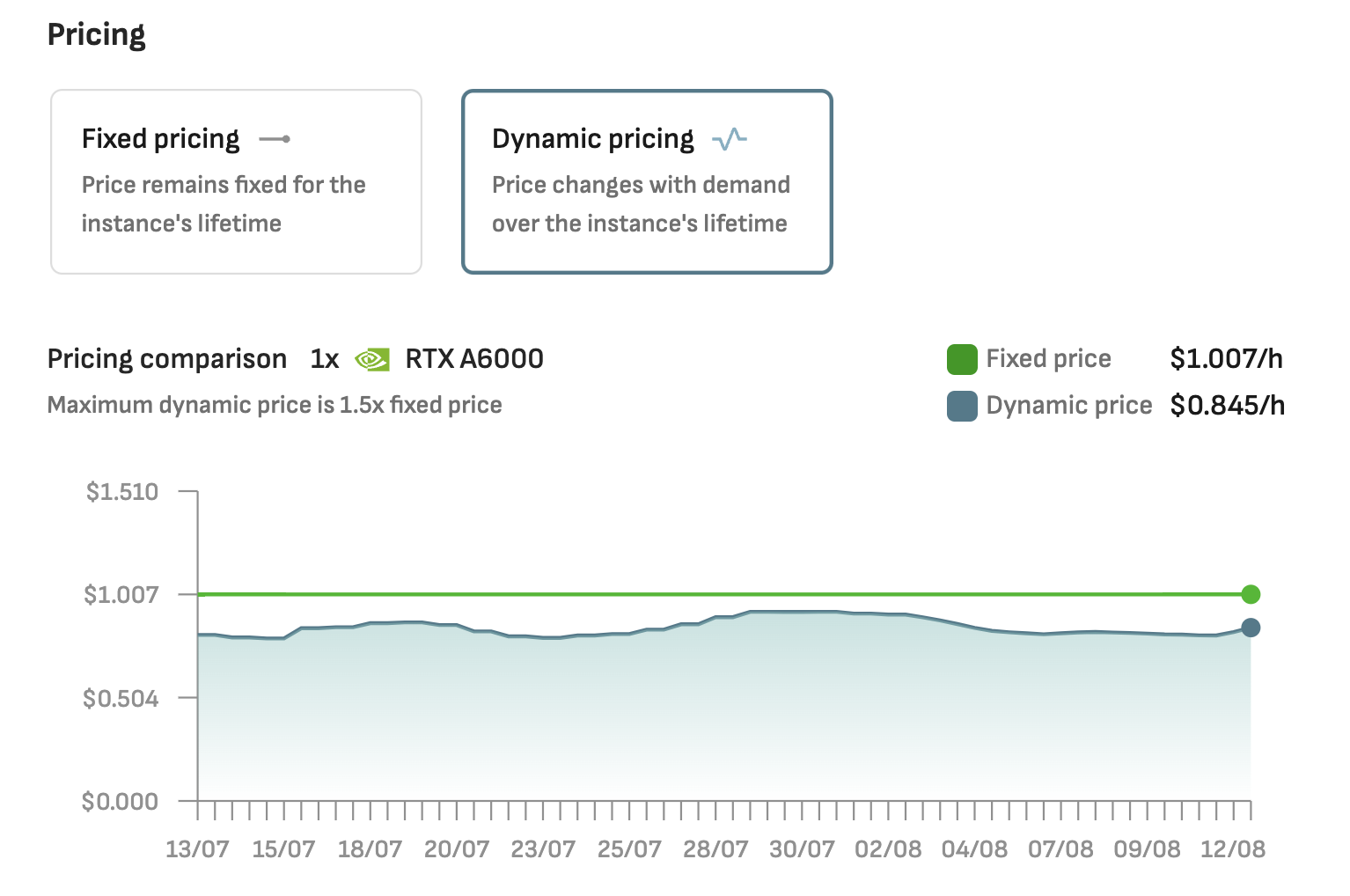
In terms of pricing, the RTX A6000 is one of the most affordable options with an approximate hourly price on the DataCrunch Cloud Platform of $1.01/h. The RTX 6000 Ada’s hourly price of $1.19/h reflects its more advanced capabilities and superior performance-per-GPU. Of the three options, the H100 comes with the highest cost at $3.17/h per GPU instance, with plenty of AI developers choosing to pay for the premium option.
Bottom line on the RTX A6000 today
Today the RTX A6000 remains a reliable, cost-effective option, particularly if you're working on medium-scale AI projects or need a versatile GPU that can handle a mix of AI and general-purpose tasks. Its cost-per-hour affordability, wider availability and power efficiency make it an attractive choice for smaller teams, startups, or individual AI engineers who are mindful of budget constraints but still require solid performance.