If you’re looking for hardware to run serious AI workloads you’re most likely choosing between different hardware options from NVIDIA. There is no other vendor out there that can give you the full performance, networking and software stack.
For larger AI training and inference projects you’ll need to consider options between building or deploying multi-GPU systems. Here you’ll run into the choice between HGX and DGX server configurations. Let’s go through some core differences and what the implications are for larger AI workloads.
What is NVIDIA DGX?
NVIDIA DGX is NVIDIA's pre-built, all-in-one AI computing platform designed for organizations that need a powerful, easy-to-deploy solution for heavy AI and machine learning workloads. Think of DGX as the plug-and-play option for enterprise businesses, where everything comes pre-configured—hardware, software stack, and diagnostic tools.
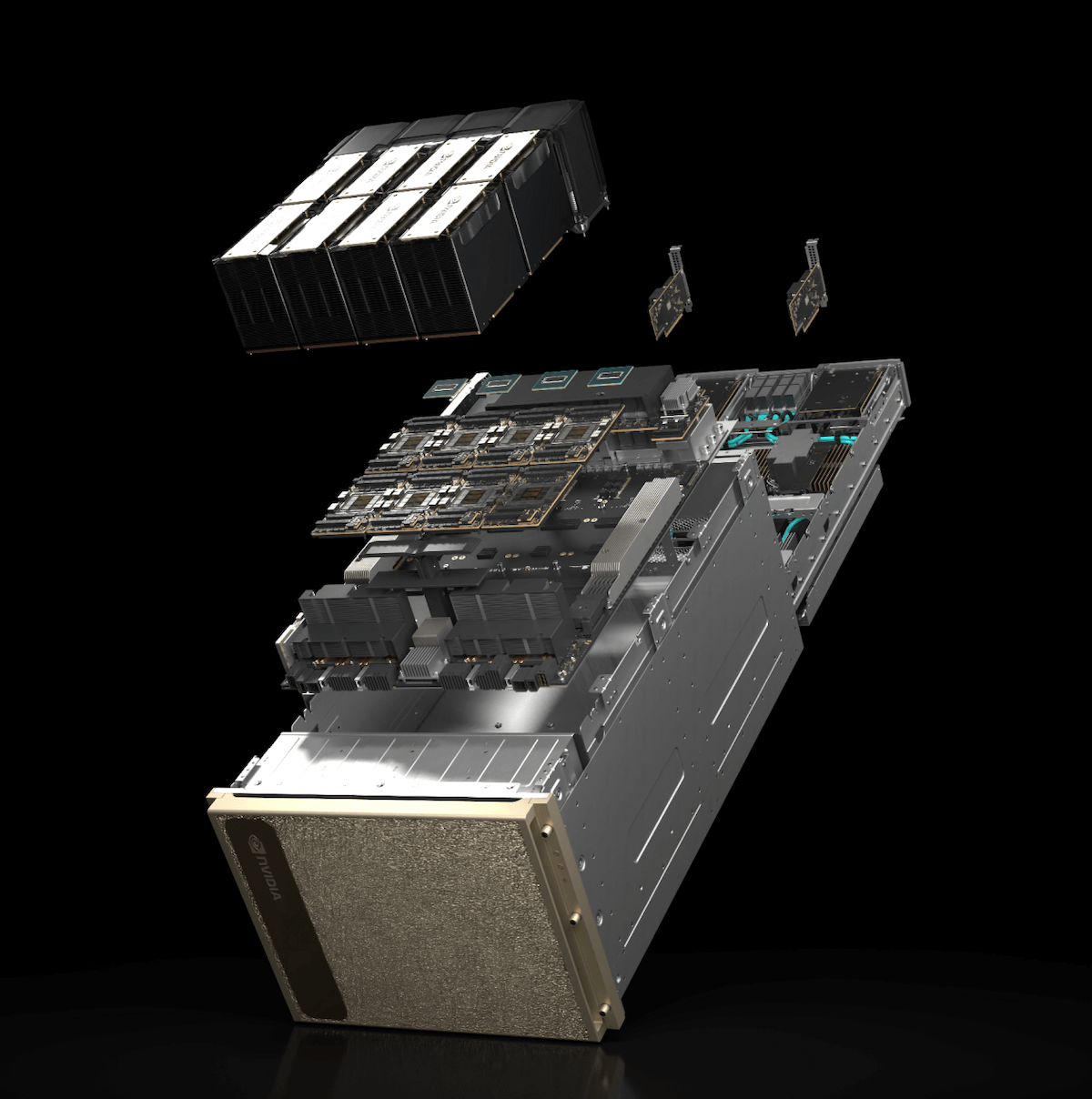
Components inside of the DGX H200 system. Source:nvidia.com
Each DGX system comes pre-installed with up to 8 NVIDIA GPUs, such as the H100 or H200, and NVLink interconnect technology for efficient communication between GPUs.* DGX supports NVIDIA’s core AI software stack, including tools like CUDA, cuDNN, TensorRT, and pre-optimized AI frameworks from NVIDIA NGC. You should think of the DGX system as unified platform combining both a hardware and software for quick deployment.
*See a more detailed comparison of the H100 vs H200 CPUs
Key Features of DGX Systems
Turnkey Solution: DGX comes pre-configured, requiring minimal setup.
Advanced GPU Configurations: Up to eight H100 Tensor Core GPUs efficiently integrated into each system.
NVIDIA Software Stack: Easy access to NVIDIA’s AI libraries, tools, and frameworks including NVIDIA Base Command, the cluster management software for DGX data centers.
Enterprise Support: DGX comes with additional services and support for enterprise businesses.
Who Should Use DGX?
DGX systems are mostly aimed at enterprise buyers. They are good if you're looking for a pre-packaged AI solution with minimal setup time and networking configuration. They could be a good fit for a research institution, a startup focused on AI development, or an enterprise with AI-driven business strategies.
What is NVIDIA HGX?
In contrast to DGX, NVIDIA HGX is not a pre-configured system. Instead, it’s a modular platform that offers you the building blocks to design and deploy scalable AI infrastructure. HGX allows you to scale performance by integrating multiple NVIDIA GPUs (like the A100 or H100) in an extremely fast and efficient way.
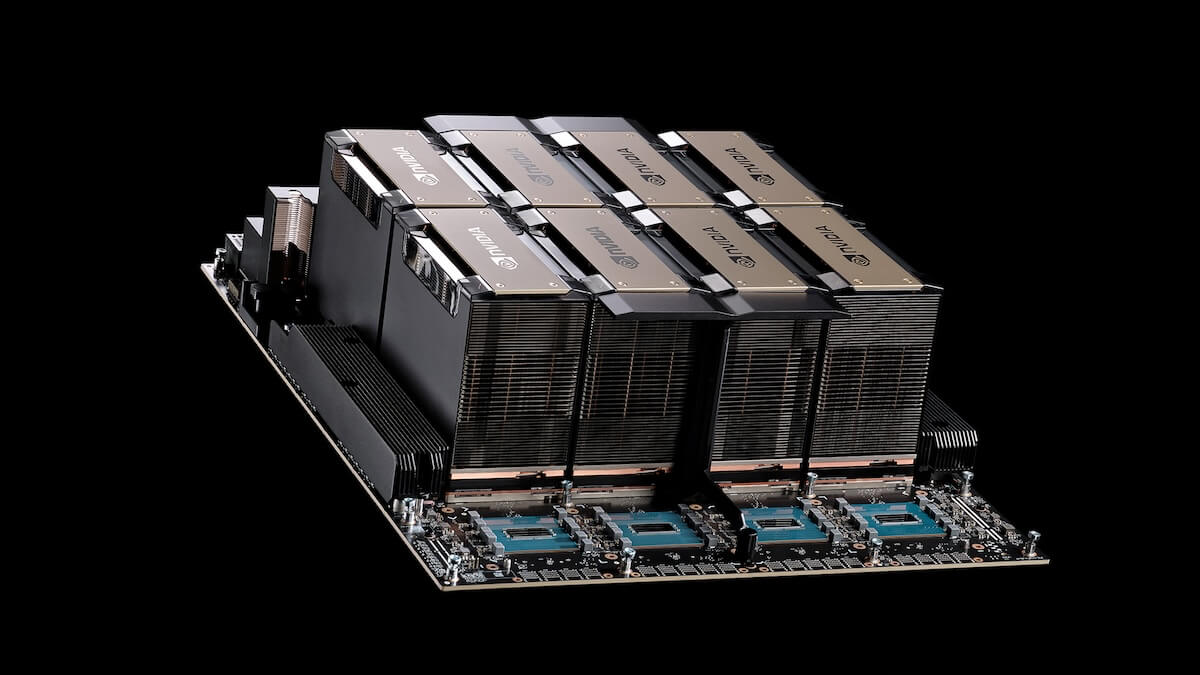
HGX H200 system with NVlink switch chips. Source:nvidia.com
HGX is tailored for datacenter requirements, incorporating advanced GPU configurations, network interconnects, and storage solutions. This means end-customers rarely buy full HGX systems, but it is an excellent option for cloud providers and large enterprises needing highly scalable, customized infrastructures for AI workloads.
Key Features of HGX Systems
Modular Flexibility: Unlike DGX, HGX doesn’t lock you into a specific configuration. You can design and optimize the system based on your performance and scalability needs.
High-Density GPU Deployment: HGX systems support massive GPU scaling, making them ideal for large-scale AI training and inference or HPC tasks.
Customizable Networking and Storage: You can choose advanced networking (e.g.,
InfiniBand, NVLink) and storage configurations to optimize for high-throughput data environments.
Data Center Integration: HGX is perfect for integrating with existing data centers, giving the ability to scale compute resources as your workload grows.
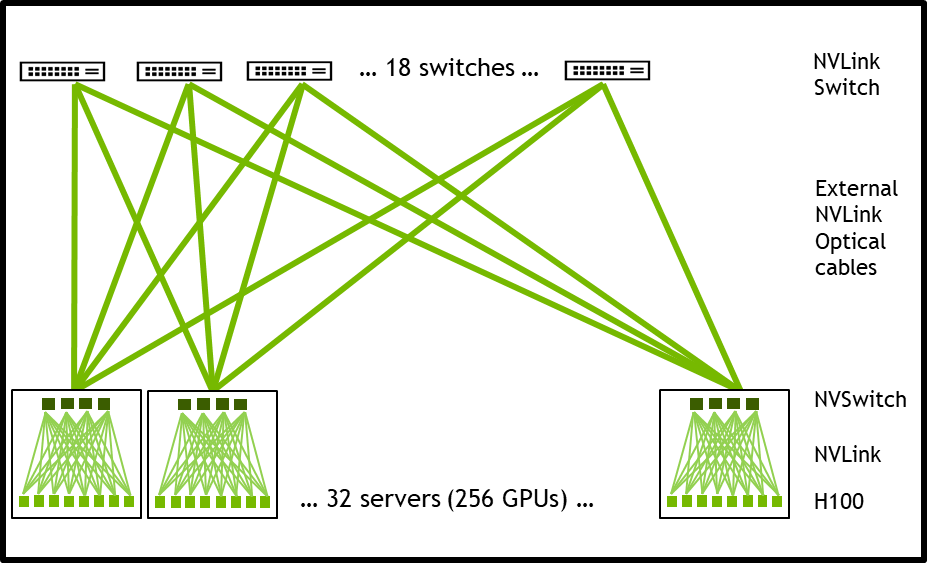
Visual representation of an NVIDIA H100 HGX system with 256 GPUs. Source:nvidia.com
Who Should Use HGX?
HGX is ideal for large-scale data center configurations. Hyperscalers, cloud providers, and large enterprises looking to create or expand large-scale HPC environments use HGX’s flexibility for adapting to specific workload demands and building out ever-larger computing clusters over time.
Technical Comparison: DGX vs HGX
Hardware Comparison
When comparing DGX and HGX, you’re essentially comparing a turnkey system versus a flexible, modular solution. Both use NVIDIA’s latest GPUs, but the deployment approach differs significantly.
DGX Systems: Typically, a DGX H100 includes eight H100 Tensor Core GPUs, connected via NVLink, allowing high-bandwidth communication between GPUs. It also includes high-performance storage and networking, all configured out of the box.
HGX Systems: You have more freedom with HGX. You can choose how many GPUs (up to 16 or more per server) to include and configure their connections (via NVLink, PCIe, or InfiniBand) to optimize for performance or cost. HGX gives you the flexibility to scale your environment as needed, whereas DGX systems are confined to predefined configurations.
Software Ecosystem
With DGX, you get a fully integrated software stack that includes NVIDIA Base Command and access to NVIDIA NGC for optimized AI containers. DGX is designed for seamless integration with NVIDIA’s AI libraries and frameworks, simplifying the process of running complex AI workflows.
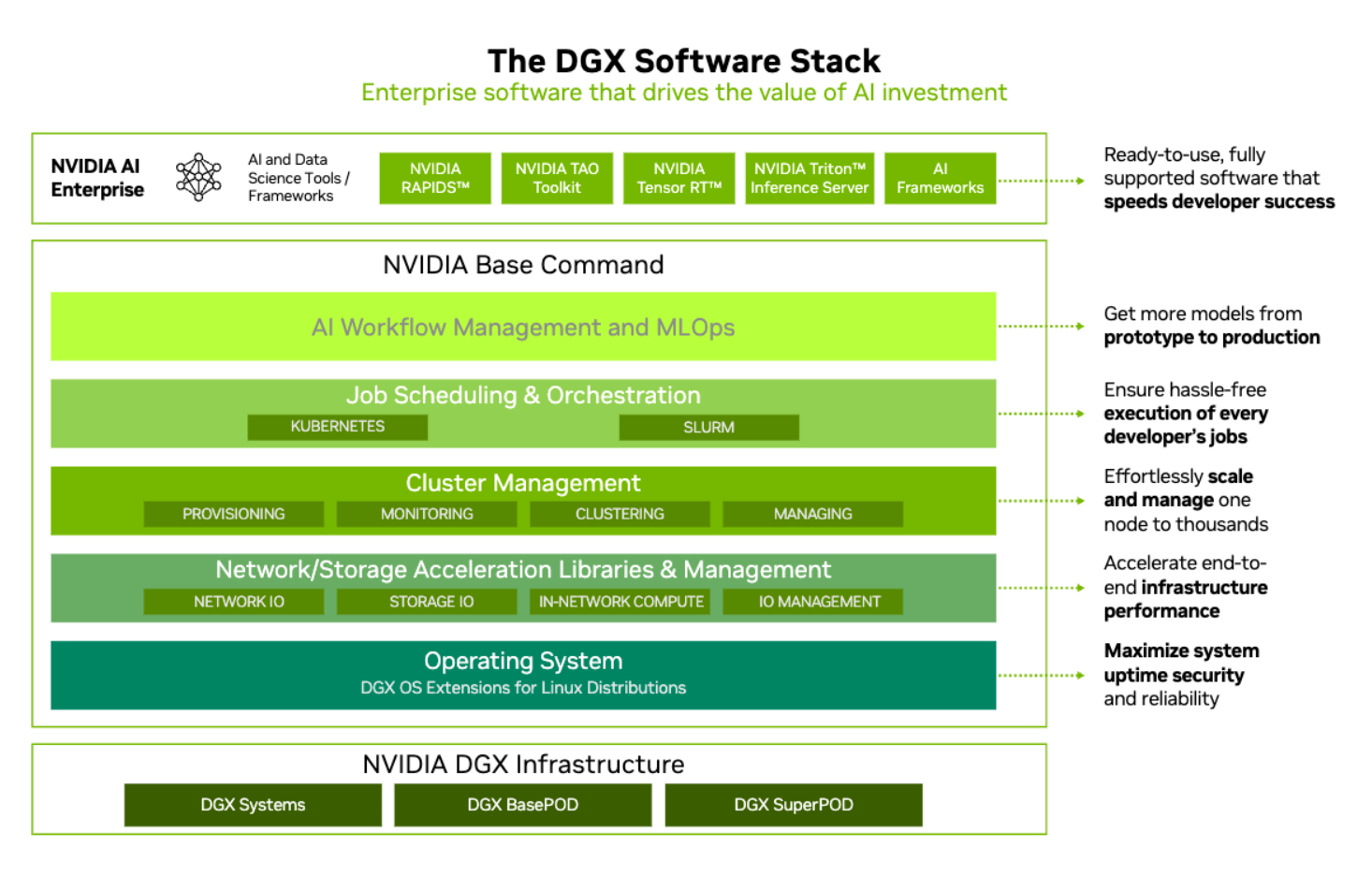
HGX, on the other hand, gives you more control over the software environment. You can integrate custom AI frameworks, orchestration tools like Kubernetes, and cloud-native services. This makes HGX better suited for anyone who requires deep customization for their AI workloads and prefer to manage their own software stack.
Deployment Flexibility
If you're a gamer you can think of the DGX like an Alienware gaming laptop. It comes pre-installed with some really good hardware and software, but you will have limited ability to make changes. On the other hand, an HGX system is like a PC gaming rig you build yourself. You'll have full flexibility on what hardware and software you use - and you can adjust over time based on your needs.
DGX: Best for single-node, immediate deployment, especially when you need to minimize setup time. You can deploy DGX systems quickly, and they are particularly well-suited for standalone research labs or enterprise AI teams with limited IT support.
HGX: Offers flexibility for integrating AI capabilities into large-scale data centers. HGX shines when you need to customize and scale your infrastructure across multiple nodes or regions.
Performance and Benchmarking
The DGX H200 and HGX H200 machines use the same H200 SXM baseboard and thus offer the exact same GPU performance, as long as the CPU and system memory are not in the picture. The CPUs and system memory are often quite impactful in the overall performance, as they are essential in feeding the GPUs.
DGX H200 | DataCrunch HGX H200 | |
---|---|---|
GPU | 8x H200 SXM5 141GB | 8x H200 SXM5 141GB |
CPU | 2x Intel 8480C - 224 threads | 2x AMD 9654 - 384 threads |
CPU relative performance (passmark) | 125165 - 100% | 233980 - 186% |
Memory | 2TB | 1.5TB - 3TB |
Memory bandwith | 306GB/s | 460GB/s |
Cost Considerations
The cost of a DGX system is straightforward—it’s a single price point for the entire system, which includes hardware, software, training and support. HGX, however, involves a more granular pricing model that depends on your choice of OEM and configuration preferences. Typically you don't buy HGX systems directly from NVIDIA. You’ll need to account for the cost of individual components—GPUs, storage, networking—as well as software and support contracts.
The big question you need to ask before choosing either option is whether you need to make up-front investments in hardware in the first place. Cloud GPU platforms like DataCrunch offer competitive hourly pricing for GPU instances and custom-built deployments of latest NVIDIA GPU clusters. In case you have doubts, you can reach out to our AI engineers directly for advice on the most efficient configuration for your needs.
Bottom line on DGX vs HGX
Choosing between DGX and HGX ultimately depends on your infrastructure needs, deployment scale, and technical resources. If you’re looking for a plug-and-play solution with limited setup and easy manageability, DGX offers a powerful and reliable option. On the other hand, if you need customization and scalability, and have the infrastructure to support a flexible AI platform, HGX is the better choice.
Deploying hardware for AI workloads is not a simple task. In most cases you’re likely to benefit from seeking a number of different options, including reaching out to cloud GPU providers like DataCrunch. Chat with our AI engineers today.