While many AI engineers’ attention has switched to newer GPUs, NVIDIA’s A100 remains a powerful and efficient accelerator for machine learning training and inference projects today.
The A100 has a solid track record and is still extremely useful for many high-performance workloads. It also has better availability in on-demand cloud GPU instances than many newer models. See a detailed review of A100 specs, price and configuration options. If you’re thinking about spinning up an A100 instance, you need to know the difference between the A100 40GB and 80GB models, as well as the performance difference between the PCIe and SXM options. Let’s go through these form factors in more detail.
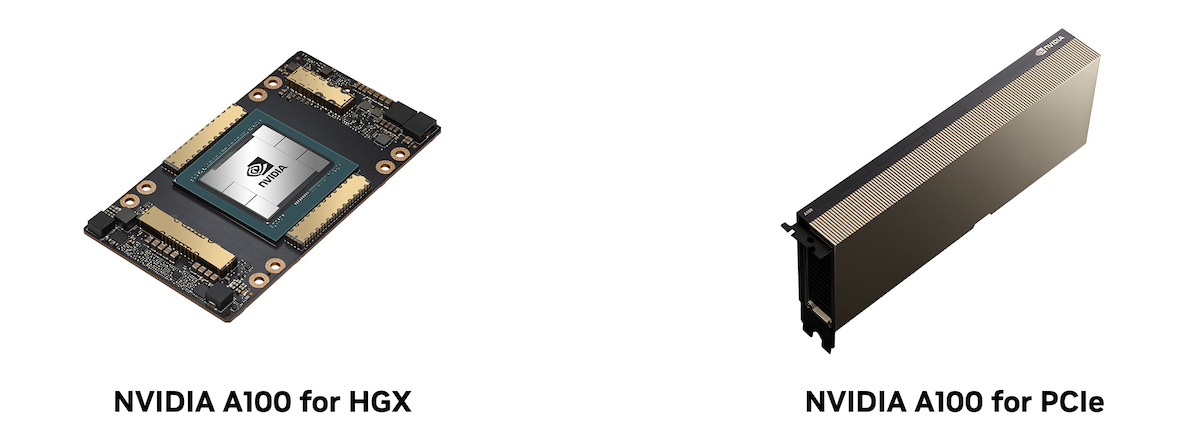
What is PCIe?
PCIe (Peripheral Component Interconnect Express) is a high-speed interface standard used to connect various hardware components to a computer's motherboard. PCI was developed originally by Intel and introduced in 1992 and the PCIe (where "e" stands for express) in 2003.
PCIe operates using lanes, which are pairs of wires that transfer data between the motherboard and the peripheral device. Each PCIe slot can have multiple lanes (x1, x4, x8, x16, etc.), with x16 being the most common for GPUs, providing the highest bandwidth.
The NVIDIA A100 uses PCIe Gen4 with 64GB/s bandwidth in a x16 configuration.
What is SXM?
SXM is a custom socket form factor designed by NVIDIA specifically for high-performance computing and intense machine learning workloads. Originally introduced with the P100 GPUs in 2016, it offers higher density and performance by integrating GPUs more closely with the system board.
SXM stands for Server PCI Express Module. It is designed to work with NVIDIA’s NVLink interconnects for direct GPU-to-GPU communication with higher bandwidth, up to 600GB/s per connection. Up to 8 GPUs can be connected in a single SXM board.
DataCrunch exclusively uses SXM technology on the A100 and H100 GPU series.
A100 PCIe vs SXM4 Datasheet Comparison
Feature | A100 PCIe | A100 SXM4 |
---|---|---|
Form Factor | PCIe | SXM |
Memory Bandwidth | 1,935 GB/s | 2,039 GB/s |
Max Thermal Design Power | 250W | 400W |
GPU Memory | 40GB | 40GB or 80GB |
Interconnect | - NVLink Bridge for up to 2 GPUs: 600 GB/s - PCIe Gen4 64 GB/s | - NVLink: 600 GB/s - PCIe Gen4 64 GB/s |
Multi-Instance GPU (MIG) | Up to 7 MIGs @ 5GB | Up to 7 MIGs @ 10GB |
When considering the implications of the PCIe and SXM form factors for machine learning training and inference projects, several key factors come into play, including performance, scalability, power efficiency, and system integration.
Performance and Speed
The SXM form factor generally provides higher performance compared to PCIe due to its superior bandwidth and power capabilities. SXM GPUs, such as the NVIDIA A100 SXM, offer higher memory bandwidth (e.g., 2,039 GB/s compared to 1,935 GB/s for PCIe versions) and higher thermal design power (TDP), allowing for more intensive computational tasks without thermal throttling. This means that for large-scale machine learning training, which often requires moving vast amounts of data quickly, the SXM form factor can deliver faster data throughput and better handle complex models, resulting in reduced training times.
SXM4 and PCIe in the MLPerf Benchmark
To illustrate the performance gap between the SXM4 and PCIe form factors, we evaluated their performance on the MLPerf training machine learning benchmark. We trained three models:
The PLM BERT-Large,
The ResNet v1.5 vision model, and
The Mask R-CNN object recognition model.
For all tasks, we used configurations with 8 GPUs, each equipped with 80 GB of memory.
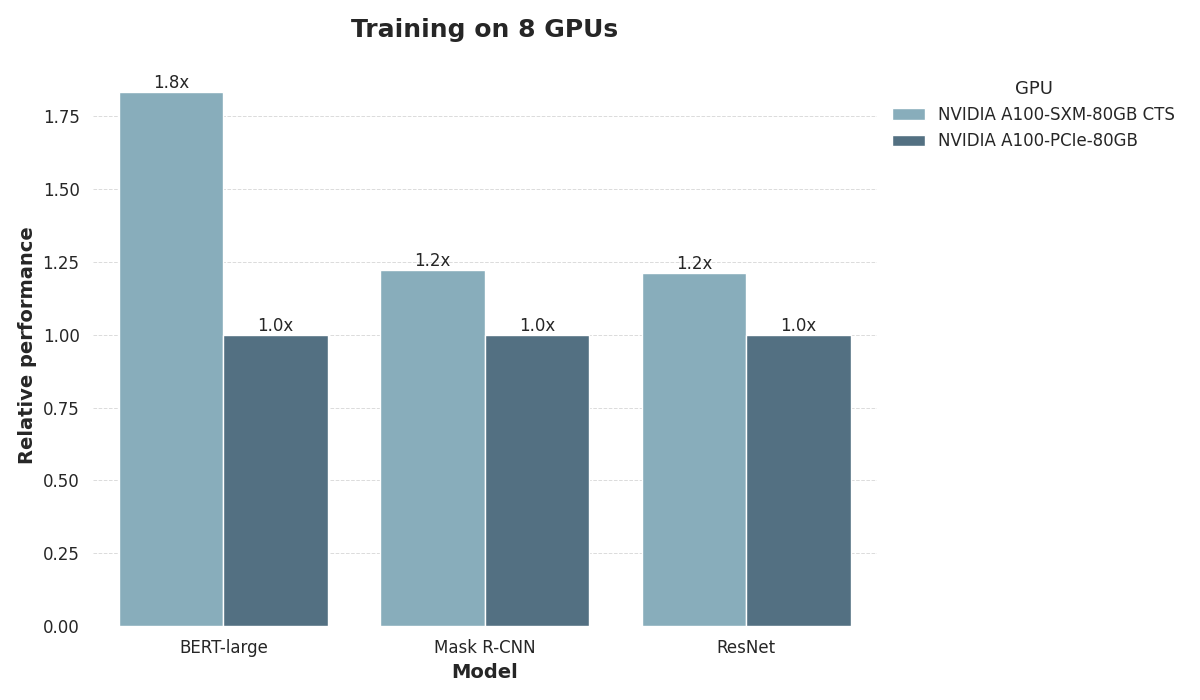
The results demonstrate that SXM4 confers a clear performance boost when training BERT-Large, where PCIe training takes nearly twice as long as training using SXM4. The performance is far more level when training the vision models ResNet and MASK R-CNN.
Scalability and Multi-GPU Configurations
SXM GPUs are designed to work seamlessly in high-density, multi-GPU configurations. They utilize NVIDIA's NVLink and NVSwitch technologies, enabling direct, high-bandwidth GPU-to-GPU communication (up to 600 GB/s per link). This is particularly beneficial for distributed training of large neural networks, where multiple GPUs need to exchange data rapidly. In contrast, PCIe GPUs, although they can use NVLink Bridges, typically support fewer GPUs with lower bandwidth, which can become a bottleneck in multi-GPU setups. Therefore, for large-scale machine learning projects that require extensive parallel processing, SXM GPUs offer superior scalability and performance.
Power Efficiency and Thermal Management
The higher TDP of SXM GPUs (up to 500W in custom configurations) allows them to sustain higher performance levels for longer periods. This is crucial for intensive training sessions that may run for days or weeks. The advanced cooling solutions typically associated with SXM modules, such as sophisticated liquid cooling systems, ensure that these GPUs operate efficiently without overheating. On the other hand, PCIe GPUs, with their more accessible air-cooled or simpler liquid-cooled designs, may be more prone to thermal throttling under sustained heavy loads.
System Integration and Flexibility
PCIe GPUs generally offer greater flexibility and are easier to integrate into existing systems, which is advantageous for smaller-scale or less specialized machine learning projects. They can be added to standard workstations or servers without requiring significant modifications or specialized infrastructure. This makes PCIe GPUs a practical choice for organizations looking to incrementally upgrade their computational capabilities.
However, if you're focused on high-performance machine learning applications, a investment in SXM-based systems can be justified by the substantial performance gains and the ability to handle more complex and larger datasets efficiently.
A100 PCIe or SXM4 for training and inference?
For training large models, especially those involving deep learning and neural networks, the enhanced bandwidth, power, and interconnectivity of SXM GPUs translate to faster convergence times and the ability to handle larger batch sizes. This can accelerate the overall development cycle, allowing researchers to iterate more quickly.
During inference, where real-time performance is critical, the lower latency and higher throughput of SXM GPUs can provide significant advantages, especially in environments where inference needs to be performed on large datasets or complex models.
Bottom line on A100 PCIe vs SXM
While PCIe GPUs offer flexibility and ease of integration, SXM GPUs provide superior performance, scalability, and efficiency for machine learning training and inference. The choice between the two will largely depend on the scale and requirements of the machine learning projects, with SXM being the preferred option for large-scale, high-performance tasks, and PCIe being suitable for smaller, more flexible deployments.
At DataCrunch we exclusively use the superior SXM4 form factor in all of our A100 and H100 on-demand instances and clusters. See for yourself the impact on raw compute power and speed by setting up your own instance today.