As new AI startups and hyper-scalers push the boundaries of what’s possible in deep learning, the demand for high-performance AI computing has never been greater.
NVIDIA’s GB200 NVL72 represents the next big leap forward in AI-focused datacenter technology. It is the next generation in immensely powerful, highly scalable, and more energy-efficient computing. For sure you’ve never seen a rack like this before.
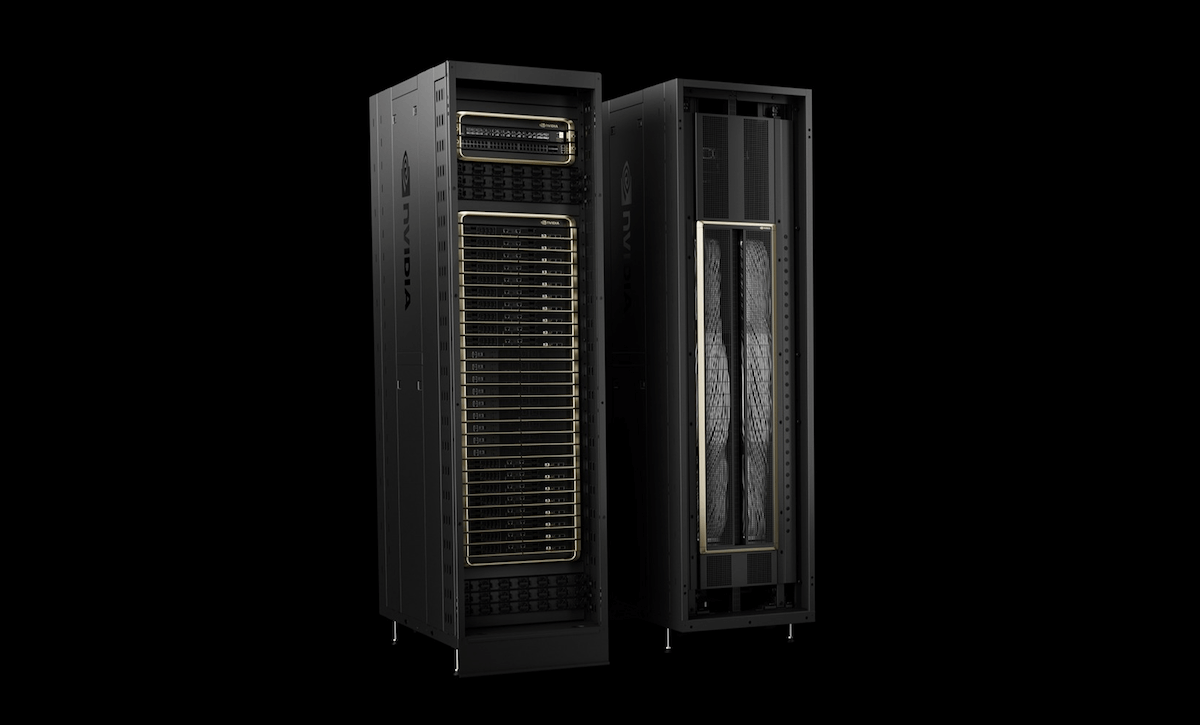
GB200 NVL72 datacenter rack. Source: nvidia.com
What is the NVIDIA GB200?
The NVIDIA GB200 NVL72 is a customized datacenter rack containing 36 Grace CPUs and 72 Blackwell GPUs connected by a 130 TB/s NVLink Switch System.
The GB200 NVL72 is designed to work as one coherent and unified GPU capable of handling the most complex AI and high-performance computing (HPC) workloads with exceptional efficiency and speed.
GB200 NVL72 Architecture
The GB200 NVL72 is powered by NVIDIA’s latest Blackwell GPU architecture, which offers a significant boost in computational power to the previous Ampere- and Hopper-architectures powering the A100, H100 and H200.
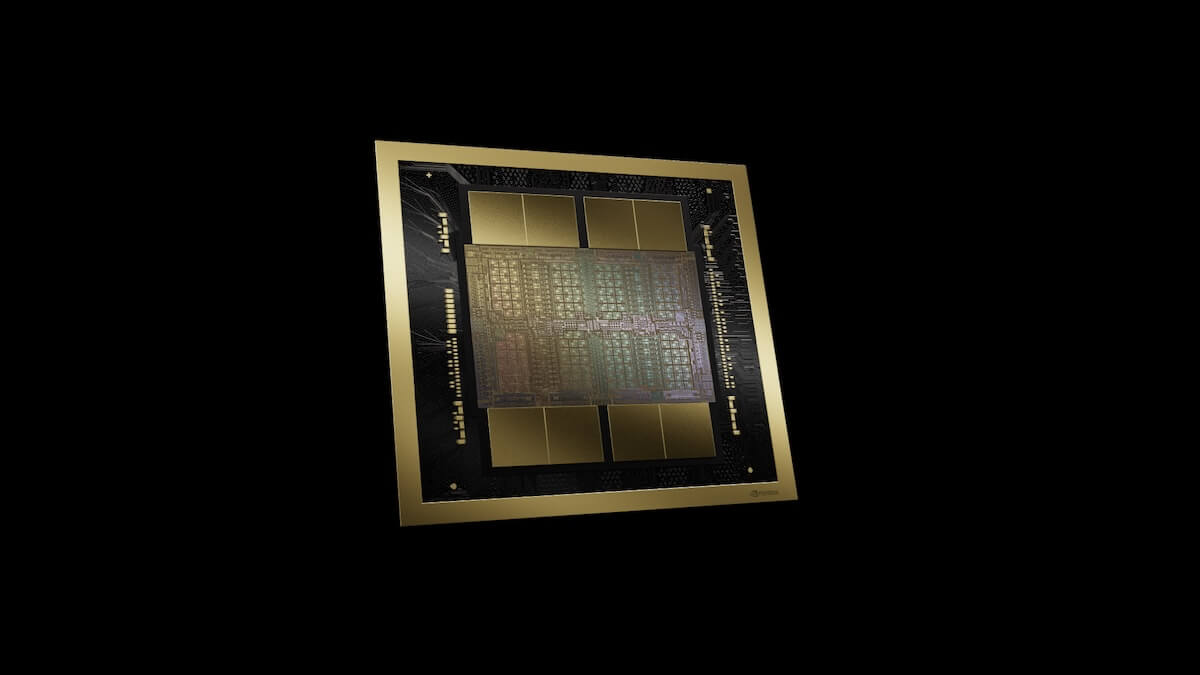
Within the GB200 NVL72 you’ll find 36 GB200 superchips where one Grace CPU and two Blackwell GPUs are set on a single die board. According to NVIDIA, each Blackwell GPU contains 208 billion transistors, more than 2.5x the amount of transistors in NVIDIA Hopper GPUs.
GB200 Networking and Memory Bandwidth Connectivity
We can expect to see four different types of networks in the GB200 NVL72 systems:
Accelerator Interconnect (NVLink)
Out of Band Networking
Backend Networking (InfiniBand/RoCE Ethernet)
Frontend Networking (Normal Ethernet)
Quantum-X800 InfiniBand is the foundation of the GB200’s AI compute fabric, capable of scaling beyond 10,000 GPU, which is 5X higher than the previous NVIDIA Quantum-2 generation. While most use AI projects won’t scale to this level, the GB200 is likely to be the benchmark to beat in datacenter GPUs for some time to come.
Blackwell GPUs include 18 fifth-generation NVLink links to provide 1.8 TB/sec total bandwidth, 900 GB/sec in each direction.
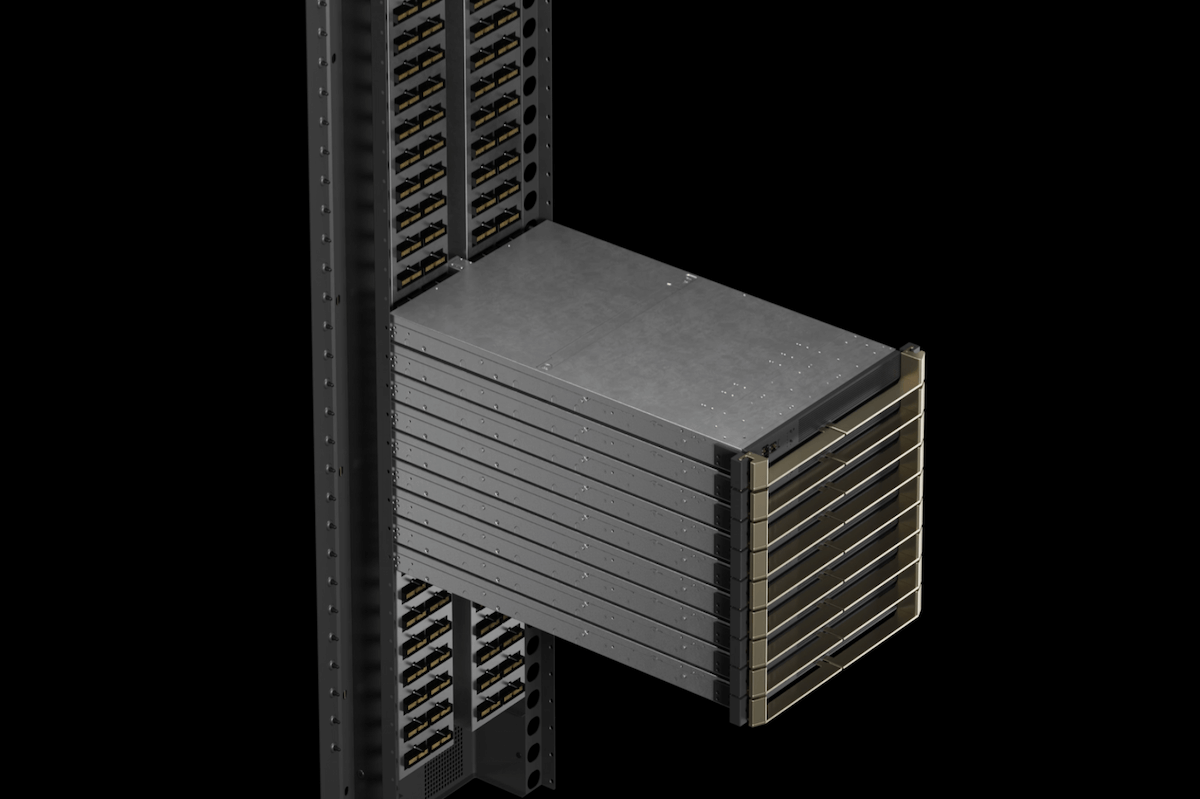
GB200 NVlink system. Source: nvidia.com
Thermal Power Management
The GB200 NVL72 is a power-hungry machine. It is likely to require 120 kW per rack, which is approximately 3x more than an air-cooled rack of H100s.
Still, the GB200 architecture incorporates several improvements in thermal power management, reducing overall energy consumption per TFLOPS. It comes with an advanced liquid cooling solution that allow it to maintain peak performance even under heavy loads.
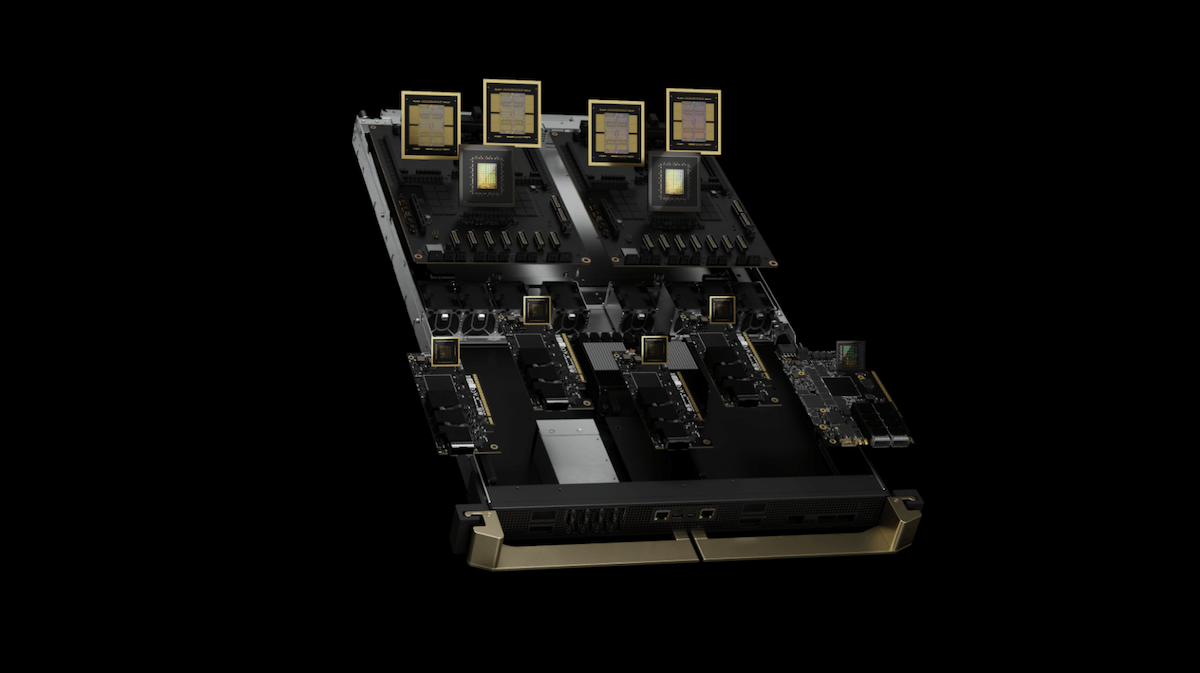
GB200 compute tray with liquid cooling. Source: nvidia.com
The per GPU power consumption of the GB200 is effectively 1200 Watts. On the whole, NVIDIA estimates the GB200 NVL72 to deliver 25X better energy efficiency at the same performance for trillion parameter AI models compared to an air-cooled H100 infrastructure.
GB200 NVL72 Specs Comparison to H100 and H200
The most natural comparison for the GB200 NVL72 is with the current highest performance NVIDIA GPUs in the market, the H100 and H200.
| H100 | H200 | GB200 NVL72 |
---|---|---|---|
Watts (Per GPU) | 700 | 700 | 1,200 |
NVLink Bandwidth (GB/s) | 450 | 450 | 900 |
Memory Capacity (GB) | 80GB | 141GB | 192GB |
Memory Bandwidth (GB/s) | 3,352 | 4,800 | 8,000 |
TF32 TFLOPS | 495 | 495 | 1,250 |
FP16/BF16 TFLOPS | 989 | 989 | 2,500 |
FP8 / FP6 / Int8 TFLOPS | 1,979 | 1,979 | 5,000 |
FP4 TFLOPS | 1,979 | 1,979 | 10,000 |
Source: nvidia.com, SemiAnalysis
*Get a more detailed summary of H100 specs and H200 specs.
AI Performance Summary
The GB200 NVL72 provides up to 30x higher throughput on AI-related tasks than the H100, especially for the dense matrix operations. Optimizations by NVIDIA help reduce latency and increase efficiency in multi-GPU configurations, leading to up to 4x speedup over the H100 in GPT-Moe-1.8T model training.
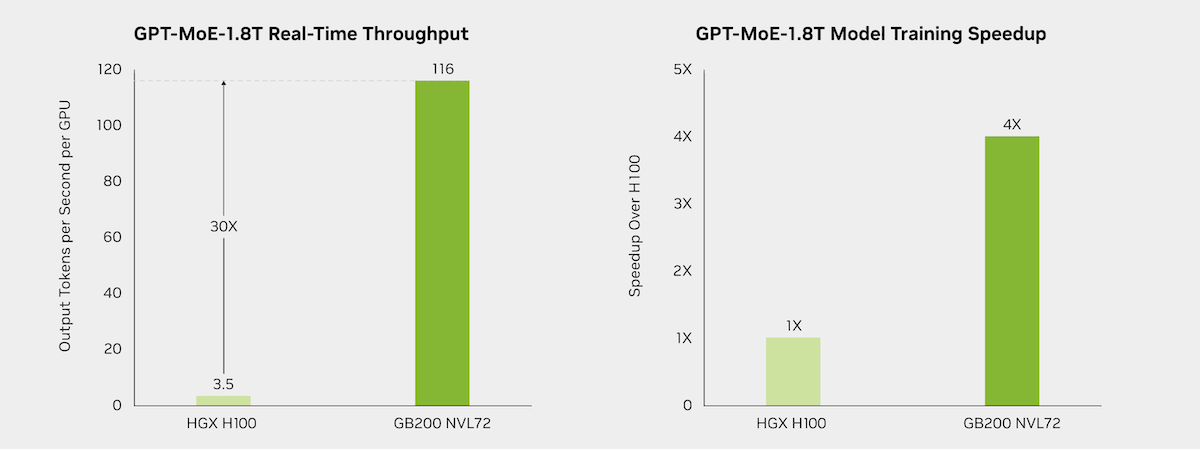
Comparison of GB200 NVL72 system with a H100. Source: nvidia.com
On the whole, the GB200 NVL72 system can do 1.44 exaFLOPS of super-low-precision floating point mathematics, making it the first exascale GPU solution.
In a real world example, OpenAI training GPT-4 in 90 days with 25k A100s. It should be possible to train GPT4- in less than 2 days with a 100k GB200 NVL72 set-up.
New Precision Capabilities with Second-Generation Transformer Engine
The GB200 NVL72 uses NVIDIA's second-generation Transformer Engine to introduce advanced precision formats, including community-defined microscaling, formats such as MX-FP6, to improve both accuracy and throughput for large language models (LLMs) and Mixture-of-Experts (MoE) models.
Micro-tensor scaling uses dynamic range management and fine-grain scaling techniques to optimize performance and accuracy, enabling the use of FP4 AI. This innovation effectively doubles the performance with Blackwell’s FP4 Tensor Core and also increases parameter bandwidth to HBM memory, allowing for significantly larger next-generation models per GPU.
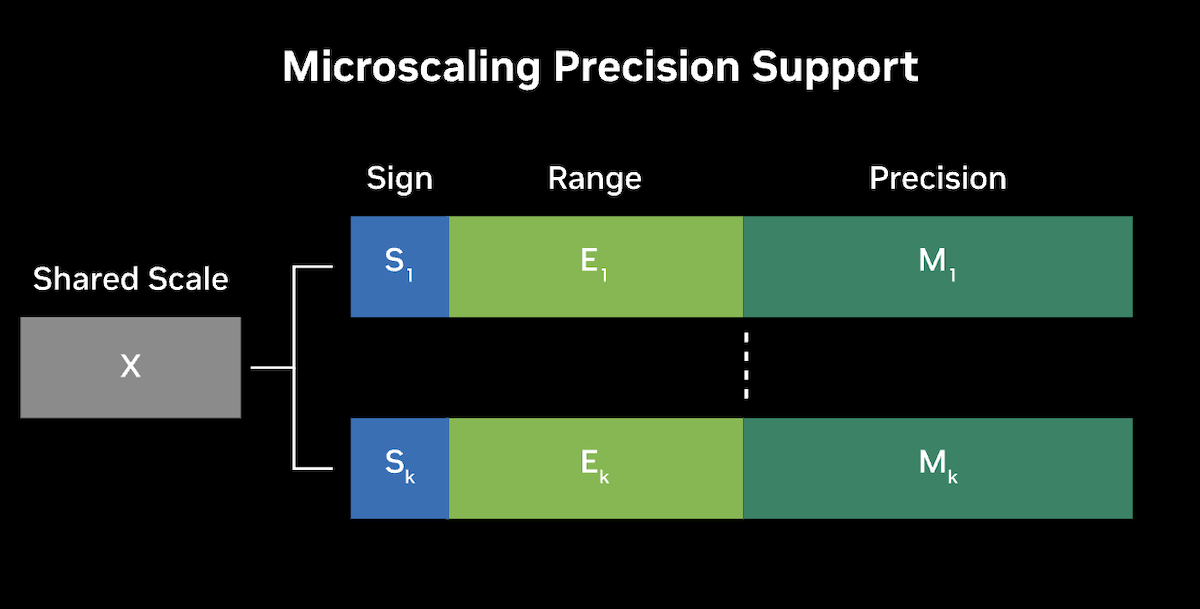
Conceptual framework for microscaling precision. Source: nvidia.com
The integration of TensorRT-LLM, with quantization to 4-bit precision and custom kernels, enables real-time inference on massive models with reduced hardware, energy consumption, and cost. On the training side, the second-generation Transformer Engine, combined with the Nemo Framework and Megatron-Core PyTorch library, provide unparalleled model performance through Multi-GPU parallelism techniques and fifth-generation NVLink support.
Bottom line on the GB200 NVL72
The GB200 NVL72 is unlike any datacenter rack we’ve ever seen. Its advancements in GPU architecture, memory bandwidth, and energy efficiency make it a powerful tool for tackling the most demanding AI workloads. For AI engineers, the NVL72 offers not only superior performance but also the flexibility and scalability needed to stay competitive in a fast-paced industry.